The role of artificial intelligence |and machine learning in IoT |
- LearnElectronics
- Dec 25, 2022
- 4 min read
Updated: Jan 6
Artificial intelligence (AI) and machine learning (ML) are playing an increasingly important role in the Internet of Things (IoT). These technologies enable connected devices to make intelligent decisions and learn from data, opening up a world of possibilities for automation, optimization, and prediction. In this blog, we'll explore the role of AI and ML in IoT and how these technologies are being used to transform industries and improve our lives.
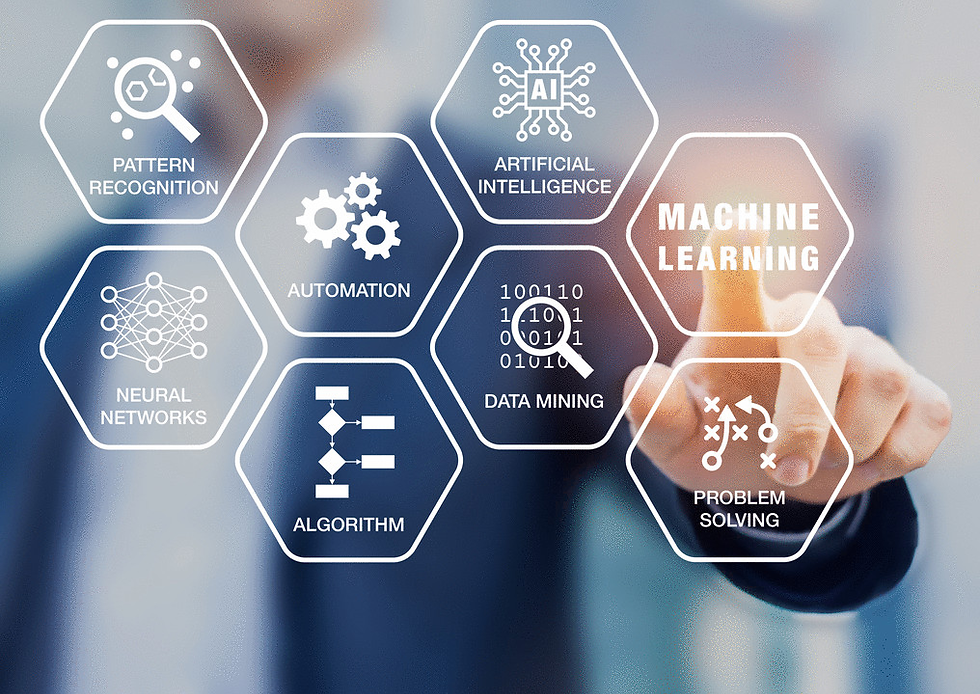
What is AI and ML?
AI refers to the ability of machines to perform tasks that would normally require human intelligence, such as learning, problem-solving, and decision-making. ML is a subset of AI that involves training algorithms on large datasets to enable them to learn and make predictions or decisions based on the data they have been fed.
How are AI and ML being used in IoT? - The role of artificial intelligence
There are many ways in which AI and ML are being used in IoT, including:
Predictive maintenance: ML algorithms can analyze data from connected devices and equipment to predict when maintenance or repairs may be needed. This can help organizations to proactively address potential issues before they become problems, reducing downtime and saving money.
Personalization: IoT devices can collect data on an individual's preferences and habits, and use ML algorithms to provide personalized recommendations or automated actions. For example, a smart thermostat may learn that you prefer a certain temperature at certain times of the day and adjust accordingly, or a smart home system may recommend products based on your previous purchases.
Fraud detection: ML algorithms can be used to analyze data from IoT devices to detect patterns that may indicate fraudulent activity. For example, a bank may use IoT data to detect unusual patterns of card usage that could indicate fraud, or an insurer may use data from connected vehicles to detect potentially fraudulent accident claims.
Predictive analytics: ML algorithms can be used to analyze data from IoT devices to make predictions about future outcomes. For example, an agricultural company may use data from IoT-enabled weather stations to predict future crop yields, or a transportation company may use data from connected vehicles to predict traffic patterns and optimize routes.
Natural language processing: AI and ML can be used to enable IoT devices to understand and respond to voice commands and other forms of natural language input. This can make interacting with IoT devices more convenient and intuitive for users.
Image and video recognition: AI and ML can be used to enable IoT devices to analyze and recognize images and video, enabling them to perform tasks such as identifying objects or people. This can be used in applications such as security systems or quality control in manufacturing.
Benefits of AI and ML in IoT
There are many benefits to using AI and ML in IoT, including:
Improved efficiency: By automating tasks and making intelligent decisions based on data, AI and ML can help organizations to operate more efficiently and effectively.
Enhanced customer experience: Personalization enabled by AI and ML can improve the customer experience by providing tailored recommendations and automating actions that align with an individual's preferences.
Increased safety: AI and ML can be used to enhance safety in a variety of applications, such as detecting potential hazards in manufacturing or predicting and preventing accidents in transportation.
Improved decision-making: By analyzing data and making predictions, AI and ML can help organizations to make more informed decisions and optimize operations.
Increased competitiveness: By adopting AI and ML technologies, organizations can gain a competitive edge by leveraging the power of data to drive innovation and optimize operations.
Challenges of AI and ML in IoT
Integrating Artificial Intelligence (AI) and Machine Learning (ML) into Internet of Things (IoT) systems brings about several challenges that need to be addressed for successful implementation.
Here are some of the key challenges:
Data Quality and Quantity:
Data Security and Privacy:
Scalability:
Real-time Processing:
Energy Efficiency:
Interoperability:
Model Interpretability:
Adaptability and Robustness:
Regulatory Compliance:
Cost:
Addressing these challenges requires collaboration among researchers, industry experts, and policymakers to develop solutions that ensure the successful integration of AI and ML in the IoT ecosystem while considering ethical, privacy, and security implications.
Check out our Free IoT Projects Playlist - IoT Projects
Order Electronics Projects
Want us to guide you through your project or make the project for you? Click on the button below or reach out to us via Call/WhatsApp at (+91) - 7600948607
You can -
Order Basic Electronics Projects
Order Embedded Systems Projects
Order IoT Projects
Order FPGA Projects
Order VLSI Projects
Order Image Processing Projects
Order Matlab Projects
Order TinkerCAD Projects
Order Proteus Projects
Click on the button below to fill out the project inquiry form -
Create Various Projects
Check out our Free Arduino Projects Playlist - Arduino Projects
Check out our Free Raspberry Pi Projects Playlist - Raspberry Pi Projects
Check out our Free TinkerCAD Projects Playlist - TinkerCAD Projects
Check out our Free IoT Projects Playlist - IoT Projects
Check out our Free Home Automation Projects Playlist - Home Automation Projects
Check out our Free NodeMCu Projects Playlist - NodeMCu Projects
Follow us -
Please do follow us i.e. #learnelectronicsindia to get daily updates about new blogs, videos, courses, products, offers, competitions, quizzes, and Internship Opportunities.
Informative and concise! LearnElectronics India always delivers quality content.
I stumbled upon this blog while researching the role of artificial intelligence and machine learning in IoT, and I must say, it's an incredible resource! The way Learn Electronics India explains complex concepts in a clear and concise manner is commendable. This article has provided me with a deep understanding of how AI and ML are revolutionizing the IoT industry. Thank you for sharing such valuable insights.